Artificial intelligence (AI) solutions hold great promise for making wastewater treatment plants more efficient and sustainable. What do plant operators think about this cutting-edge technology? To find out, we conducted a survey, measuring user acceptance of AI in wastewater treatment.
Assessing user acceptance
Our online survey garnered responses from 10 participants across various European wastewater treatment plants. Most of the survey participants were directly involved in day-to-day operations of the treatment plant. They had different levels of experience, ranging from 1 to 33 years. Interestingly, most participants were already familiar with AI systems that either provide suggestions or make automated decisions in the wastewater treatment plant. While this familiarity is helpful, it also poses challenges in managing biases from their prior experiences.
In the survey, we presented participants with three concrete scenarios on how the treatment plant could function with varying levels of AI involvement. For each scenario, we asked participants to rate the confidence, enjoyment and likelihood of adopting the technology. Then, we evaluated their responses using the Technology Acceptance Model (TAM). This is a theoretical framework for understanding and predicting the acceptance and adoption of new information technologies by end users.
Balancing automation and human oversight
We found no significant differences in how useful or easy-to-use participants found the three different scenarios. This implies that they saw value in all of them. However, there was a notable trend in confidence, enjoyment, and willingness to adopt: confidence and enjoyment was lower in scenarios where the AI was more autonomous. These systems were less likely to be adopted. This highlights that finding a balance between automation and human oversight is crucial.
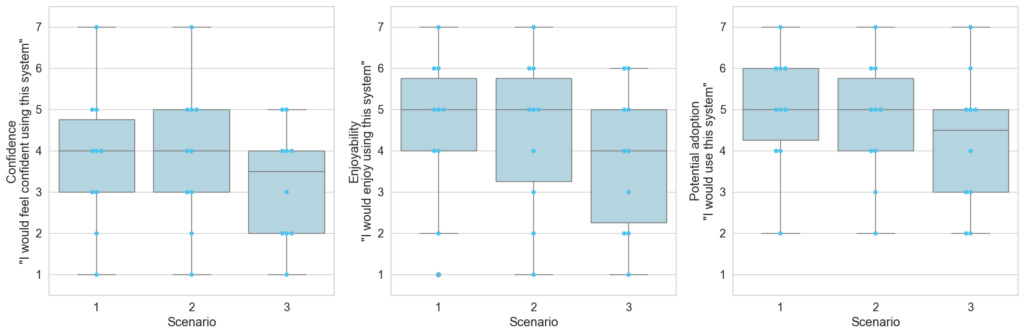
Survey results showing operator confidence (left plot), enjoyability when using the system (middle plot) and likelihood of adopting the system (right plot). Scenarios vary in AI involvement: Scenario 1 has the lowest AI involvement, scenario 3 the highest.
Building trust with Explainable AI
Explainable AI techniques emerged in our survey as critical for fostering trust in AI systems among human operators. The “black-box” nature of AI algorithms can create mistrust, as users find it challenging to understand the rationale behind recommendations. Explainable AI helps human operators understand why the AI makes certain choices, for example by showing which factors influenced a decision or visualising how the AI model works.
Our survey also highlighted the importance of providing clear information about expected outcomes, reliable feedback mechanisms and clarity on consequences. Furthermore, the survey brought to light concerns regarding AI training, system reliability, and company policies. This highlights areas that require further investigation and improvement.
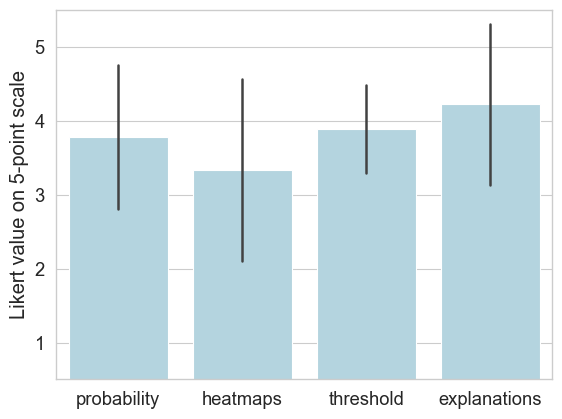
Wastewater treatment plant operators showed a high preference for explainability, with the highest preference for textual explanations. Preference was measured on a 5-point scale.
User-Centric AI Solutions in Wastewater Treatment Plants
The survey results underscore the importance of user acceptance and transparency when implementing AI in wastewater treatment plants.
Based on our results, we devised the following guidelines to increase user acceptance and trust for AI solutions in wastewater treatment:
-
Transparency
Clear communication about how AI generates recommendations and the data sources it relies upon is crucial for fostering trust.
-
Accuracy and consistency
Consistently accurate recommendations build trust over time and reinforce users' confidence in AI systems.
-
Explainability
AI systems that offer explanations for their recommendations help users understand and trust the decision-making process.
-
Human-AI collaboration
Involving users in the development process and allowing them to provide feedback can enhance their sense of ownership and trust.
While our findings provide valuable insights, further research incorporating controlled experiments and mock-ups is needed to validate and enhance our understanding of what operators want and need.
Ultimately, the journey towards harnessing the full potential of AI in wastewater treatment plants requires a collaborative effort. The goal is to develop user-centric solutions that prioritise transparency, usability, and the symbiotic relationship between AI systems and human operators.